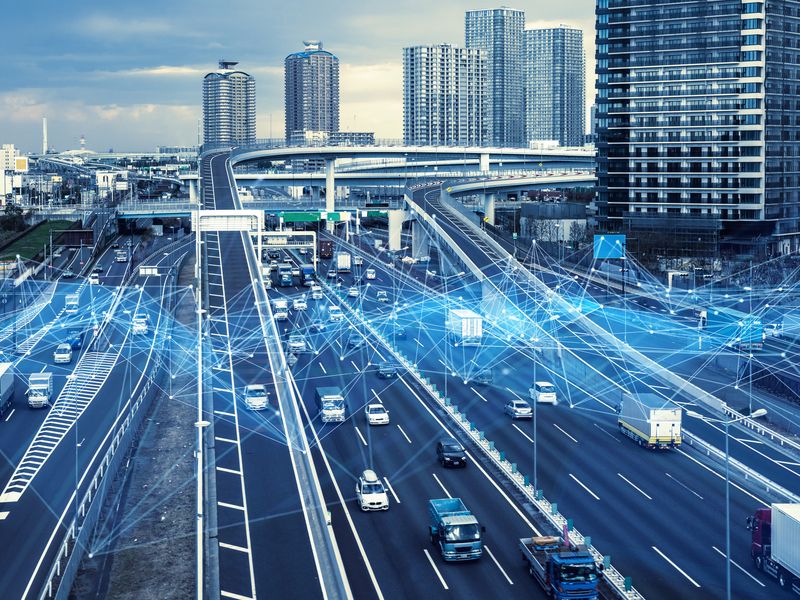
The past two years have brought a torrent of challenges for the automotive supply chain. Even beyond Covid-19, there have been blocked canals, congested ports, driver shortages and multiple natural disasters that have surprised OEMs and suppliers around the world. The pandemic exacerbated these issues and created new ones, most notably a critically restricted supply of semiconductor chips.
A key culprit is making these challenges worse: outdated software.
“If you’re reacting to a supply chain crisis with old and inflexible software written in the 1990s, it’s a bit like playing a game of chess where you can only see some of the pieces,” says Liam Mawe, Global Head of Automotive and Mobility at Palantir Technologies. “Data and analytics in the supply chain should light up the entire chess board and guide decision-makers in the supply chain to make moves with the full available context towards the strategic goals of the company.”
For many business leaders today, it’s becoming increasingly clear that data technology needs to bridge the gap between analytics and operations. Companies like Palantir lead the way by integrating data in common operating platforms designed for operational decision-making. This creates creating environments where supply-chain crises can be managed intelligently with contextual detail or prevented altogether.
This approach is part of a broader push to revolutionize the automotive industry supply chain by introducing new tools harnessing the power of data and AI technology. Two of the key avenues towards making this happen: leveraging enterprise-wide data and the power of artificial intelligence (AI).
According to Mawe, data tends towardentropy. There are potentially billions of nodes to map across a supply chain and many of them can be concealed in siloed departments or dated pieces of software, both of which hinder transparency.
“The antidote to this challenge is an enterprise-wide semantic layer that effectively translates raw data into a recognizable language for end-users,” says Mawe.
Palantir calls this common language an “ontology,”and it’s the first step towards building a digital twin of an enterprise, a simulation that allows decision-makers to understand complex systems within their organizations. By integrating siloed data systems, Palantir creates a common operating picture from which users can run AI models, test scenarios and prevent problems before they happen.
According to a study by McKinsey & Co., a global consulting firm, “Successfully implementing AI-enabled supply-chain management has enabled early adopters to improve logistics costs by 15 percent, inventory levels by 35 percent and service levels by 65 percent.”
Mapping the supply chain gives end-to-end, real-time visibility into disruptions, strategically reduces risk and can present a robust set of levers to pull when crises do occur. The power of cloud computing and machine learning means supply-chain data and possible reactions to deviations can be implemented in an almost real-time, automated way, rapidly reacting to disruptions.
Properly mitigating risk and responding to crises requires building useful and relevant analytical models from the ground up. How companies go about building them has a major impact on their potential success.
“In the supply chain world, there are a myriad of different data sources, such as enterprise research planning (ERP) systems, transport management systems (TMS), warehouse management systems (WMS), shipping notices, spreadsheets and desktops,” says Mawe. “All of that data needs to be captured and modeled to provide true end-to-end visibility.” Perhaps counterintuitively, however, the best place to start is with modeling real-world situations for procurement managers and other employees on the ground level.
Software integrations can quickly draw the data out of disparate silos like ERP and WMS systems and put them into a digital twin of the organization. But it’s still not a rapid or easy process to integrate all this data while tracking managerial decisions.
“Supply chains are a balancing act, because an improvement in one area might have negative downstream effects on another,” Mawe says. “That necessitates a shared language for how users report their data and how they access it later on, a lexicon referred to as a ‘uniform semantic layer.’”
According to Mawe, by starting with employees in real scenarios rather than high-level data collection, “You get much better adoption of analytical solutions because you’re meeting the users where they work.”
Once data, decisions and operations are properly mapped into a single system, users can run AI-informed simulations to model complex scenarios. These scenarios are then communicated clearly via digital assistants and dashboards to decision-makers, laying out the potential consequences of a decision. Faced with a critical component shortage, for example, managers can use these dashboards to intelligently decide whether to reallocate, change suppliers or shipping methods or halt production.
After decades of just-in-time production methods, there’s very little slack in the supply chain, so data visibility and rapid decision-making can be the difference between finding supply or being beaten to it.
“What stakeholders need most in that situation is a rapid breakdown of the implications of decisions,” Mawe says. “You might have cost, profit, quality, speed of resolution, which customers are affected, what are the environmental implications and so on.”
The algorithms are there to help people make better decisions, he adds. And after decisions are made, they can be fed back into the models to help them learn for the next set of potential scenarios.
Traditionally, the most difficult part of mapping a supply chain is identifying all the different silos and sidings where pertinent information may be stored.
“With automotive, you could spend your entire life trying to model the entire supply chain and all the global implications,” says Jafaar Beydoun, sales director at software firm o9 Solutions. “For something like EV batteries, you could get all the way to lithium mining.” It’s possible to model all those relationships in time, he says, but it’s better for companies to focus first on their most vital partners and suppliers.
“One of our clients was using our software for supply-and-demand predictions, but they realized that without getting the suppliers directly involved, their information was always outdated,” Beydoun says. “To get real-time information in one place, they figured out the most critical and highest-spend suppliers and first integrated the ones they worked best with first, then adopted suppliers farther down the list later on.”
Mapping this way is iterative and after perfecting the onboarding and data exchange processes with the first set of 15 suppliers, it’s easier to extend it to the next group of 10 or 15, all the way down to the smallest suppliers. As an example, Beydoun cites a small supplier in Bangladesh that did not have a reliable internet connection, but which could upload a single weekly data sheet to inform forecasting models.
“This OEM reached almost total supply-chain visibility in two years with this phased approach, about half the time it would have taken if they’d tried to implement all vendors at once,” he says.
Both o9 Solutions and Palantir use Amazon Web Services (AWS) as their cloud-computing platform, which allows them to spin up computing power as needed. But AWS’s expertise comes into play in other ways, too.
“Many organizations are reconfiguring their IT needs around the platforms that Amazon Web Services provides,” says Manish Govil, the company’s supply chain global segment leader. “We have the ability to gather and organize data from disparate systems, such as point-of-sale, ERP and Internet of Things (IoT) devices.” AWS’ data ingestion, transmission and storage pipeline provides the capability to stitch together data from those disparate systems for end-to-end visibility.
The company also has plenty of expertise managing its own supply chain, with many partners who have highly specialized capabilities in different areas of the supply chain. “We understand demand-shaping, sensing and planning, transportation management, real-time transportation visibility and warehouse management,” Govil says. “There are a lot of organizations that can provide one or two of these areas of expertise, but we have a very extensive ecosystem that brings them all together.”
Along with Apple, Proctor & Gamble, McDonald’s, and Unilever, Amazon is one of five companies deemed a “supply-chain master” by consulting firm Gartner, best known for its annual list of the top 25 supply-chain management companies. “That experience informs how AWS helps other companies build similar types of systems,” Govil says. “There are already business networks that have built the connective tissue for supply-chain visibility through AWS.”
While the data is owned by the individual companies, this connectivity allows very specific data to be shared from many disparate players far faster than any conventional data-gathering process – and speed is of the essence.
During the course of the past two years, and throughout several disruptive “black-swan” events, the industry is undergoing a mentality shift. “Many OEMs and suppliers got caught flat-footed by the pandemic and the chip shortage and they’re now looking to lay out scenarios three, six or nine months down the road that can help them better manage the state of the world,” says Jason Clark, VP of Industry at o9.
Data from suppliers can map out a universe of possible options for parts substitutions and supply-line shifts. “There are only so many combinations and you can model out the rules for how components will flow pretty precisely,” Clark says. “When you hit a black-swan event or major disruption, that’s where the AI can proactively recommend solutions or implement them, almost in real-time.”
Based on accumulated knowledge from past experiences and a supply-chain map, “an algorithm can identify the solution that has the least impact on your objective, whether that’s on-time customer delivery or margin contribution or whatever. Specific objectives like this can be programmed into the AI and monitor historical performance across the network and variability across each of the nodes,” Clark says.
This makes organizations more agile and resilient. Furthermore, the recurrence of patterns also informs organizations about the relatively small number of key levers that have the most impact on business and what pulling one more of those levers will mean for the bottom line. “For the highest-impact decisions, the levers are easy to understand, but machine learning can really help with the unusual outliers,” Beydoun adds.
In the wake of COVID-19, Clark says there’s been a knee-jerk move away from historical just-in-time supply-chain methods to more of “just-in-case” situations. “But I think they’ll swing back more towards just-in-time methods when they’ve got more time to do enough additional analysis to take on acceptable risks,” he says.
“The chip shortage added a level of volatility that OEMs and suppliers haven’t seen in a long time and the creative solutions people have come up with are here to stay,” Clark adds. That includes better forecasting and reducing the time it takes to respond to market shifts from months to weeks to even days.
“Some OEMS are further along than others,” Beydoun says. “This level of urgency needs to kind of permeate throughout the procurement and planning organizations. Data makes this possible,” he adds, “but you also need people and processes working in concert with the tools.”
With chip supplies still short after almost two years of trying to remap supply, and with more COVID-related lockdowns on the way, OEMs are feeling the pressure to adapt and to wrangle more of their data. By harnessing the power of data, and bridging the gap between operations and analytics, they can come closer to ensuring enterprise stability even during the most challenging of supply-chain crises.