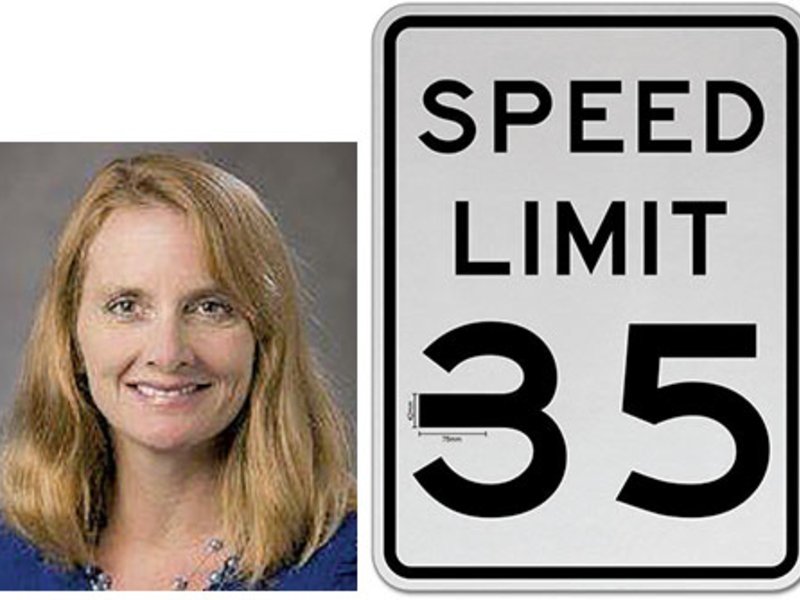
Billions of dollars have been spent in a global race to develop artificial intelligence in recent years and position AI as a powerful technology capable of redefining multiple industries.
Might some of those efforts be unraveled by a small piece of black tape?
A recent experiment underscored the fragility of nascent artificial intelligence systems. Researchers at McAfee tricked a Tesla equipped with Traffic-Aware Cruise Control into misreading a speed-limit sign and then accelerating to match the erroneous reading.
They altered a 35 mph speed-limit sign by elongating the middle part of the “3” with a patch of black tape. The tweak baited Tesla’s computer-vision algorithms into misclassifying the “3′ as an “8.”
For now, this may be of mild concern. The spoofed system is part of a driver-assist feature. Humans still oversee — and retain all responsibility for — vehicle operations. But it’s not a far leap to a future when similar algorithms govern the actions of self-driving systems, and that portends trouble for not merely Tesla, but for an entire industry.
The McAfee research is only the latest display of “brittleness” in artificial intelligence systems, says Missy Cummings, a professor at Duke University and director of the school’s Humans and Autonomy Laboratory and Duke Robotics. She says machine learning, a subset of AI that’s in this case used to train computer-vision algorithms such as the one in the Tesla, isn’t yet all that smart.
“There’s no ‘learning’ going on here,” she said. “If we mean that I see a set of relationships and then I can extrapolate that to other relationships, I would call that learning. All machine learning does is recognize and make associations that aren’t always correct.”
Inferring contextual information that may be beneficial or critical to safe operations is an area where AI struggles. It cannot yet reason or adapt to uncertain circumstances.
“A human driver would see that ‘3’ with the extended middle line and know that something was up,” Cummings said. “You’d say ‘God, that’s a terrible new design. I wonder what DOT was thinking?’ Or if you saw an 85-mph speed limit in an urban area, you’d know kids had been screwing around. You’d understand that the speed limit is still 35.”
This month, she published her own research on the shortcomings of machine learning. In her paper, “Rethinking the maturity of artificial intelligence in safety-critical settings,” she argues that while AI holds promise for assisting humans in operating systems, this should not be mistaken for the ability of AI to replace humans.
Such a conclusion comes at a pivotal junction. The auto industry has stopped short of selling active systems that can claim driving responsibility from humans. Indeed, there’s been a proliferation of enhanced driver-assist systems reaching the market, such as Tesla’s Autopilot. But that dividing line between human responsibility and computer responsibility is where rubber meets reality.
As Cummings suggests, part of the problem lies within the way machine learning “teaches” itself. These systems must ingest vast volumes of data that often must be labeled by humans. Unless a system sees every conceivable permutation of a particular scenario — an elongated middle line on the number 3, for example — it’s hard to make assurances that any system is without risk. Shades of lighting. A shrub that obscures a full sign. A missing piece of a sign. A pedestrian walking a bicycle across a road. All sorts of variables can confound a system that has been trained on a finite amount of scenarios. Companies preparing to launch self-driving systems may say they simply need more examples of a particular condition to train their systems.
“While that is one answer, it begs the question as to how much of this finger-in-the-dyke engineering is practical or even possible,” Cummings wrote in the Duke paper.
This “bottom-up reasoning” that demands massive amounts of data from cameras may work well in controlled environments, but it remains a barrier for using machine learning to make sense of computer vision in safety-critical fields such as transportation.
Concerns with machine learning’s maturity raise broader questions about how software gets implemented in the transportation realm, where any vulnerabilities come with the potential for deadly consequences. Two fatal crashes involving Boeing’s 737 MAX serve as a stark reminder of what happens when immature software is integrated into a mature hardware such as a vehicle, Cummings said.
As Congress reignites its efforts to pass legislation that may pave the way for self-driving vehicles to reach the road en masse, Cummings says it is important for legislators to be cognizant of AI deficiencies. The McAfee research may provide a breakthrough moment.
“People get that,” she said. “You could read any number of papers on adversarial machine learning. But for people who feel like we’re moving too fast on the legislation — and I am one of those people — this will be a good illustration. I do think this will have an effect.”