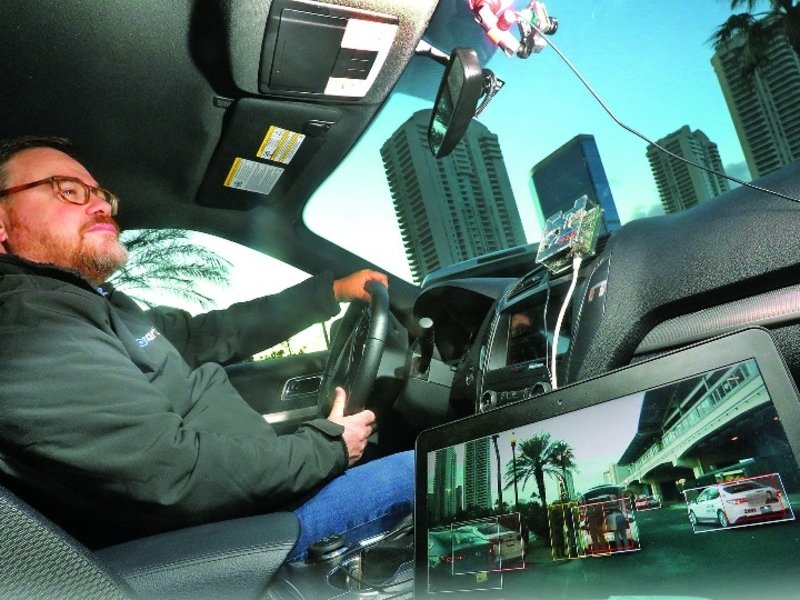
Some of the smartest engineers and academics around the world have spent years pioneering artificial intelligence and seeking breakthrough advances.
Maybe they should leave the work to toddlers.
That’s the premise of Cartica AI, an Israeli company taking a childlike approach to rethinking artificial intelligence. Rather than conventionally train machine-learning systems with reams of information, the company’s technology trains systems by mimicking the way the human brain develops.
“If you are a newborn and you look into this world, you have no idea what you see,” Karl Thomas Neumann, former Opel CEO who is a board member and investor in the company, tells Automotive News. “But you start structuring things — lines, curves, relationships.”
Cartica calls those simple structures “signatures,” and with remarkably few of them strung together, the company believes AI systems can make intelligent inferences about what’s in images captured by cameras. Such technology could play a role in improving the performance of driver-assist and self-driving systems.
Toyota AI Ventures and BMW i Ventures, the venture capital arms of the respective automakers, along with supplier Continental, invested in the Tel-Aviv based startup’s Series B funding round last September. Cartica, a spinoff from a more general-purpose AI company, Cortica, has raised $70 million since its founding last year.
What interests the automakers is the technology’s knack for using those signatures to make intelligent and accurate inferences when spotting new objects — or familiar ones in unusual positions. For example, all systems must identify all stop signs. But it becomes more difficult if the stop sign is partially obscured by shrubs or snow.
Historically, companies collect data from on-road testing and go through the laborious and expensive process of having human workers label thousands of images captured by cameras. That data is fed into the neural networks used in machine learning.
Images of every conceivable scenario are needed to teach the systems. Some companies now believe, as they get closer to identifying almost all those scenarios, the task of preparing for autonomous cars actually gets more difficult because even more data is required to root out the ever-rarer “edge cases.”
Cartica argues its unsupervised machine-learning methods can skip those problems because its signatures can make the proper inferences with minimal information, sometimes as little as one signature.
“This is how the brain works, by clustering,” said Cartica COO Barak Matzkevich. “Human beings don’t need years to know something. … Deep learning is coming to a reckoning. Can you train for all those edge cases? If not, you are restricted to highway driving.”
Cartica is not the only company eyeing an unsupervised learning approach. But its particular technology is underpinned by more than 200 patents, the company says, and it appears on the horizon at a time when self-driving leaders and others are growing more skeptical about traditional AI approaches and their suitability for autonomous-driving technology.
Last month, Starsky Robotics founder Stefan Seltz-Axmacher wrote a detailed and frank reflection on the sudden demise of his startup and his concerns about the broader shortcomings of the self-driving industry. At the top of his list: “Supervised machine learning doesn’t live up to the hype,” he wrote. “It isn’t actual artificial intelligence akin to C-3PO. It’s a sophisticated pattern-matching tool.”
One month earlier, security researchers showed artificial intelligence-based systems could be easily manipulated into misclassifying speed-limit signs. Missy Cummings, a professor at Duke University and director of the school’s Humans and Autonomy Laboratory and Duke Robotics, questioned the competence of machine-learning systems.
“There is no ‘learning’ going on here,” she said in February. “… All machine learning does is recognize and make associations that aren’t always correct.”
Whether Cartica’s self-described “self-learning technology,” which utilizes its own unsupervised method, can provide an antidote to the shortcomings of supervised maching learning remains to be seen. But it has an added benefit. Matzkevich says the platform uses about 10 percent of the power of traditional systems. Power consumption remains an important, if underappreciated, consideration for automakers.
He says the company has been working to integrate its platform with a global company this year, and there are aspirations that Cartica’s technology could be in series production by the end of 2021. The first iteration of the software will be targeted at the driver-assist market while work on self-driving tech will come down the road.
In both scenarios, Neumann — a seasoned executive from his days with Opel, Volkswagen and Continental — said he believes the systems have potential to change the industry and road safety significantly.
“When I saw this, I thought it was too good to believe, and I was very skeptical,” he said. “I said, ‘What do you do with edge cases?’ and they said, ‘With this, you don’t have edge cases.’ I’ve been looking at this idea of autonomous driving for 28 years going back to a Volkswagen project. This is a new approach.”